
We will not share your e-mail address with any third party, and we will only use it for updates to MRST. If you provide an e-mail address we will notify you when a new major version of MRST, or a critical bugfix for the current version is released. If you have questions, feedback, or suggestions, please contact Register for updatesĪs noted in the terms of usage, we ask that you register your country/affiliation to let us better evaluate the usage of MRST. The first release, designated MRST 1.1, published in April of 2009.
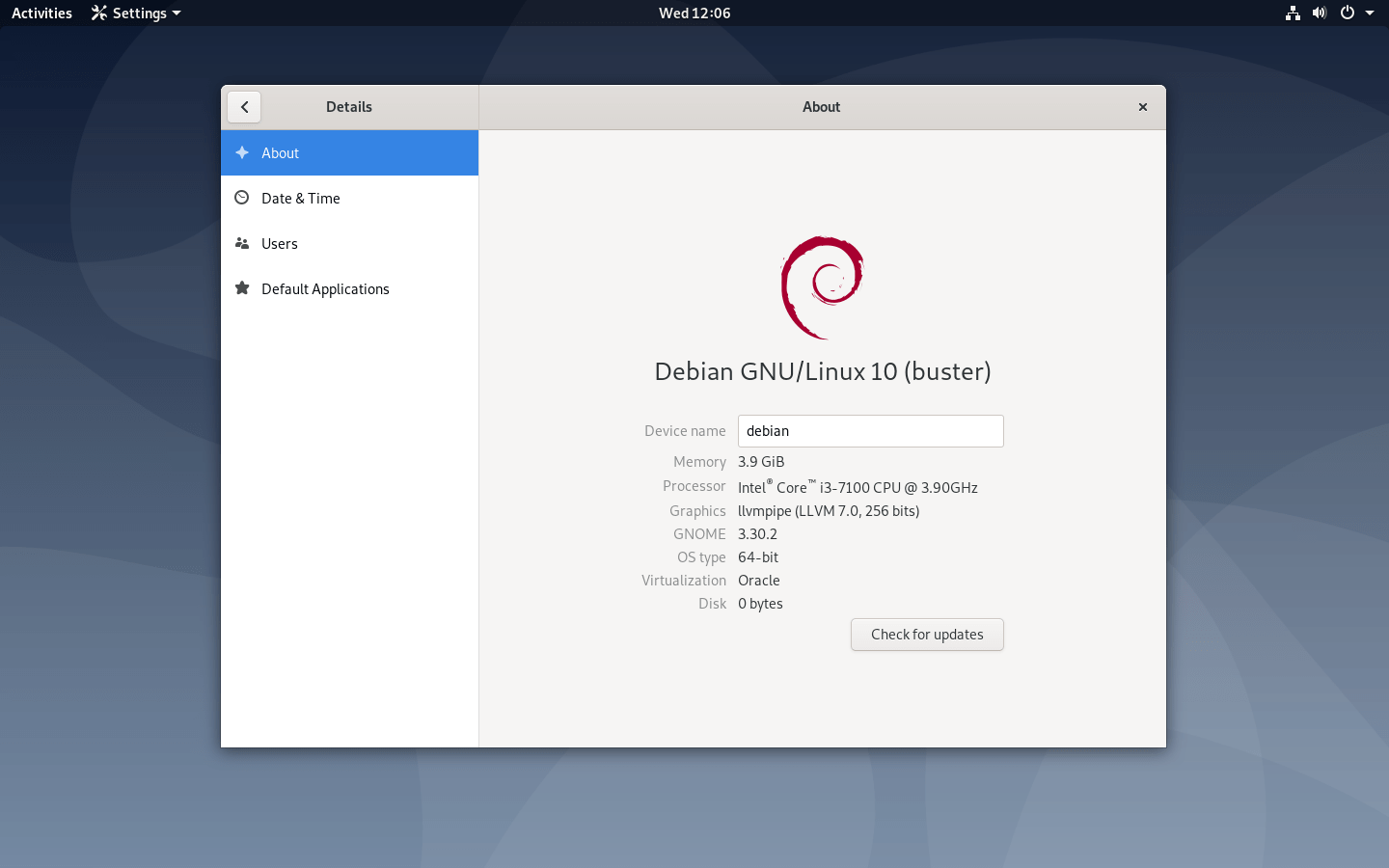
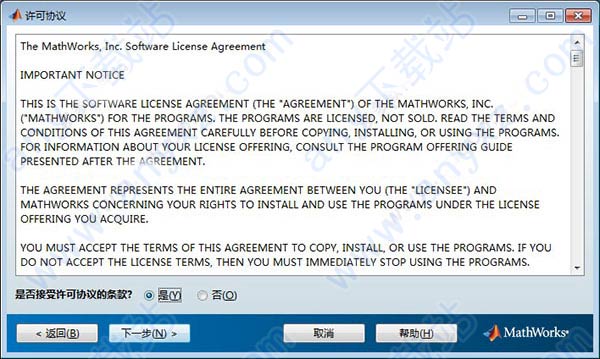
and other system requirements are provided with the tool documentation. The 14th release, MRST 2016b, published on the 14th of December 2016. The SSD Toolbox was designed in Matlab 2018b and requires the Matlab Compiler.The 15th release, MRST 2017a, published on the 15th of June 2017.The 16th release, MRST 2017b, published on the 21st of December 2017.The 17th release, MRST 2018a, published on the 1st of August 2018.The 18th release, MRST 2018b, published on the 20th of December 2018.The previous release, MRST 2019a, published on the 28th of June 2019.
Matlab 2018b system requirements software#
In particular we publish the following software packages These editions are unmaintained, however, and we encourage our users to employ the current release if possible. The release manager verifies that the MRST software does run on the same MATLAB version however (e.g., that MRST 2018a runs on MATLAB 2018a).įor backwards compatibility, we also publish the sources to earlier editions of the MRST software. Note that while MRST releases are named using a scheme that is similar to the MATLAB software from The MathWorks, the naming does not reflect minimum requirements on the MATLAB version with which it is used. Unless you have specific reasons not to, you should generally choose the most recent version of the package which can be found here. Shell leverages all those techniques and tools so that its engineers can easily and painlessly use the latest findings.The most recent edition of MRST was released on the December 16th 2019. MATLAB Production Server lets the team create thin web clients that operators in the field can use, with minimal physical hardware such as a smartphone. With minimal setup, MATLAB Distributed Computing Server™ allows the team to train networks on multiple remote GPUs in the cloud. James and Amjad will show how MATLAB ® make using these techniques easy. Automatic tag detection in large (panoramic) plant images also leads to more efficient maintenance. In geomatics, terrain classification can be improved using a rich training dataset of labelled satellite images. Shell and the Advanced Analytics Center of Excellence (AACoE) are using these techniques to speed up processes while increasing their reliability. Machine learning and deep learning can be used to automate a range of tasks.

How MATLAB ® Distributed Computing Server™ and Machine Vision Tools Are Transforming Shell
